At Sift Healthcare, we talk to many providers who think they’re using data science because they’re using automation in their revenue cycle. And without investigation, that sounds right. But, “automation” does not mean data science. The term “automation” can refer to any number of automatic processes within your revenue cycle workflows. But, just because a process is automated doesn’t mean any data science is at play. That said, here is my attempt to understand the difference between automation and data science.
Segmenting Denials
A significant misunderstanding when it comes to automation and the revenue cycle workflow is around segmentation. Segmentation is the separating denials (or patient payers) into different groups or workflows so you can work them the most effective and intelligent way. Your process for working a high-dollar denial likely differs from how you work a denial from Payer X or a denial that’s about to age-out. To approach denials with this in mind, they need to separated so that the teams working them take the correct approach. Most EHRs (or ARMs) provide a way to segment denials (and patient payers) automatically. However, this automatic segmentation is rudimentary — it is a simple rules-based process and it does not involve data science.
Rules-Based Segmentation
For most healthcare providers, denials are automatically sorted and prioritized based on dollar amount and age/date. This segmentation, rules-based segmentation, is accomplished through rules that have been set up within your EHR. These rules are thresholds that are based on predetermined *human* assumptions and they are likely limited to two dimensions. Your denials are segmented by only two attributes: dollar amount and age/date.
While rule-based segmentation of denials happens automatically, it is not driven by data science. Rules-based segmentation doesn’t allow the data itself to reveal the most meaningful differences between denials. The same two dimensions always sort your denials. There is no adjustment when external factors change, like when a Payer changes their rules or a plan is modified. And, while dollar amount and account age seem logical, restricting your view and strategy to these two dimensions is limiting. There are a host of determinants that impact if a denial will be overturned and how much will be collected. Very often, the denials with the highest dollar amounts are the ones least likely to be overturned.
Rules-based segmentation is the standard approach for denials management, but it is constrained. A better way to sort denials is based on their likelihood of being overturned. Sorting in this way requires data science, specifically, predictive analytics…
Predictive Analytics
Predictive analytics uses your own historical data to segment your denials — data around denial type, CPT code, payer, dollar amounts, etc. — and its relation to overturn success metrics. Predictive analytics use cluster analysis to identify similar groups of denials by finding the smallest variations among denials within different segments. All of the variables that impact payment outcomes are examined, and the most important are surfaced and used to segment the denials.
This is scientific segmentation. It is not driven by human assumptions. Predictive analytics are based on actual outcomes and identify the denials that are most valuable to your organization. Results correspond to the direct truth of the data. Sorting denials in this way enables you to work them more strategically and efficiently.
Predictive analytics equip healthcare providers to measure results based on outcomes, allowing them to identify how different dimensions (payers, facilities, CPT codes, etc) impact payment. And, this approach is dynamic — segments adjust every time the predictive model runs.
Using data science (predictive analytics) to prioritize denials can have an immediate impact on denial rate, overturn rate and cash flow. And although not likely to come from your EHR, predictive analytics can be integrated into the EHR and the revenue cycle workflow to optimize denials management.
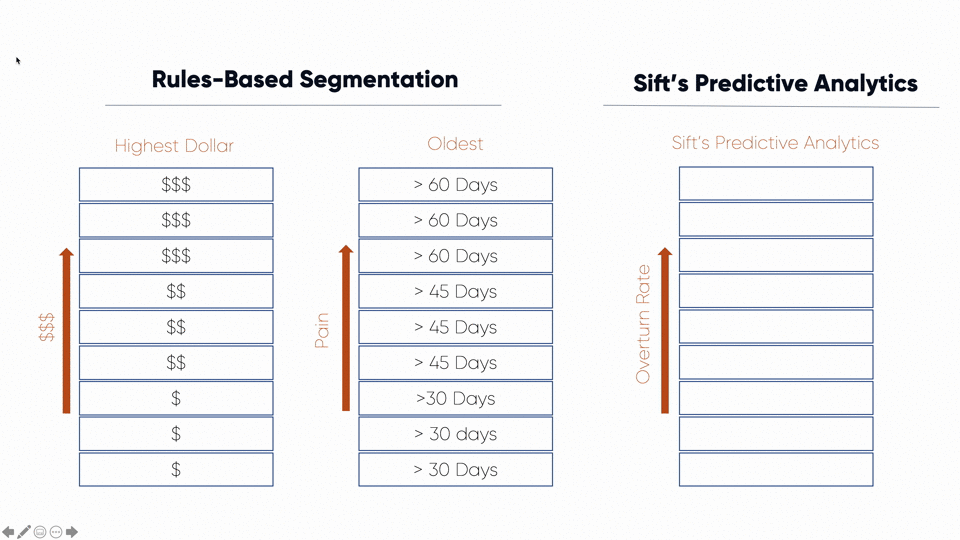
The De-Buzzed Takeaway
The summary: An automated approach to denials management does not equate to data science. Most automation in the revenue cycle workflow is rules-based, which again, is not data science.
But, data science can be (and should be) used to help prioritize denials. This involves applying predictive analytics which allows your own data to speak. Your data determines how your denials are prioritized. Predictive analytics are a more custom and strategic way to approach denials management. It's automation with a side of data science. See how Sift does it, here.